Machine Learning (ML)
Machine Learning (ML) is an approach to artificial intelligence that combines statistics and data science to develop and apply algorithms that improve their output through experience without being explicitly programmed to do so; in other words, algorithms that can "learn" to detect patterns, make decisions, and predict outcomes.
In it's simplest form, machine learning consists of several inputs, called features, a model, and an output that represents some sort of prediction.
(prediction)"))
An example of this is a spam filter: It takes inputs of an email's headers, subject, and body and determines whether the message is spam or not. A machine learning approach to spam detection will automatically learn new patterns with new data, making it difficult for spammers to defeat the filter except in the short-term. This is more efficient than traditional programming in which each rule would have to be developed by hand in response to new patterns as they emerge, allowing for longer periods between the emergence of a new pattern and a solution to detect it.
What are features?
Inputs to a machine learning model are called features and consist of statistical data types. Types of features include:
- Numerical features, sometimes called quantitative features are numbers. The n umbers may be either discrete (e.g. the number of votes in an election) or continuous (e.g. the volume of water in a glass).
- Categorical features, also known as qualitative features, consists of descriptive data that does not have a mathematical meaning. For example: Gender, color, and favorite food are all types of categorical data. Qualitative/categorical features are input into models using one-hot encoding.
- Ordinal features are a mix of categorical and numerical data, where the data fall into numerical categories. For example: a 5-points scale for product reviews.
Machine Learning Terminology
Word | Definition |
---|---|
Data sampling | Systematic creation of smaller representative samples of larger data sets |
Feature | A variable with high relevancy to the outcome variable |
Feature selection | Automatic detection of variables most relevant to the outcome variable |
Imputation | Correction of corrupt and missing values through inference |
Integer encoding | Assignment of an integer value to a categorical value, e.g. values "red", "green", and "blue" could be assigned integer values of 1, 2, and 3 respectively |
One-hot encoding | Assignment of a bit-mapped binary value to a set of categorical values, e.g. a "color" category with potential values of "red", "green", and "blue" could be mapped to three bits of 100, 010, and 001, respectively |
Outcome variable | The value to be predicted by a Machine Learning Model |
Outlier | A observation significantly different from other observations of the same data |
Overfitting | When a model performs well on training data but does not generalize well when the model encounters new data |
Regularization | Simplification of a model to avoid overfitting |
Underfitting | When a model performs poorly because it is too simple. The reverse of overfitting. |
Additional terminology can be found on types of machine learning.
The Machine Learning Process
Machine learning resources
Deeper Knowledge on Machine Learning (ML)
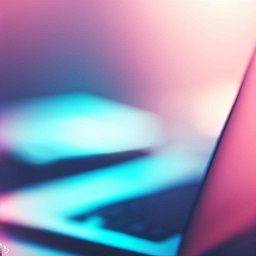
Machine Learning Project Outline
An outline and checklist to guide typical machine learning projects
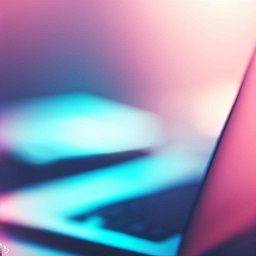
Types of Machine Learning
An overview of the types of machine learning
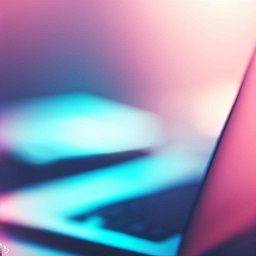
SMOTE: Synthetic Minority Oversampling Technique
Synthetic Minority Oversampling Technique: An approach to compensating for severe class imbalance in machine learning
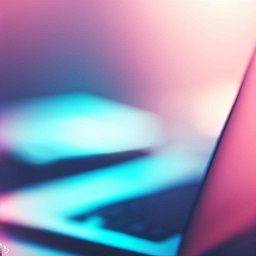
Python Open-Source Machine Learning Libraries
Python libraries used for machine learning
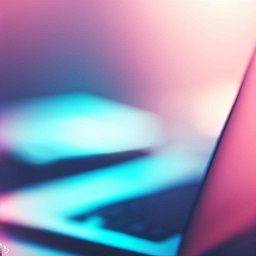
Data Mining
A guide to finding patterns and relationships in data
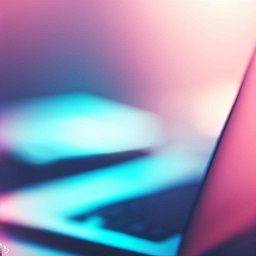
Data Wrangling
Transforming "raw" data into a more easily analyzed form through normalization and format standardization
Broader Topics Related to Machine Learning (ML)
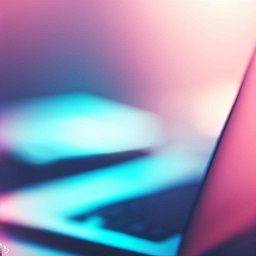
Artificial Intelligence (AI)
The mimicking of human cognitive functions and behaviors by machines
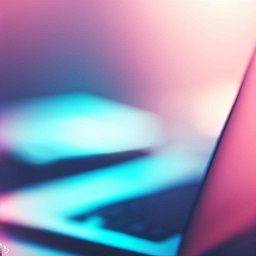
Data Science
The scientific method applied to data analysis